From the Pinnacle Blog
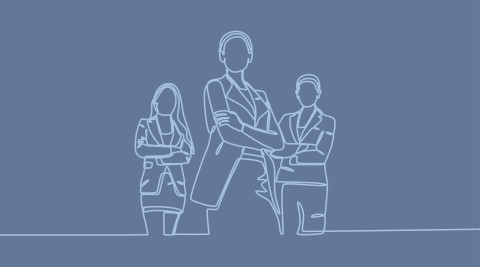
Challenging Gender Norms in Work and Life
Read how Megan Baker challenges social norms as a working mother.
Read Now
3 minutes
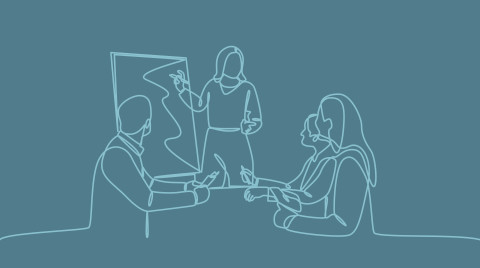
Building Confidence and Breaking Barriers
Read Nicole McArdle's advice for fostering confidence as a woman in actuarial consulting.
Read Now
2 minutes
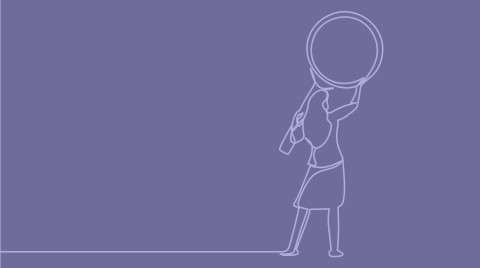
A Journey in Leadership
Read Laura Maxwell's experiences as a women in leadership in honor of Women's History Month.
Read Now
2 minutes
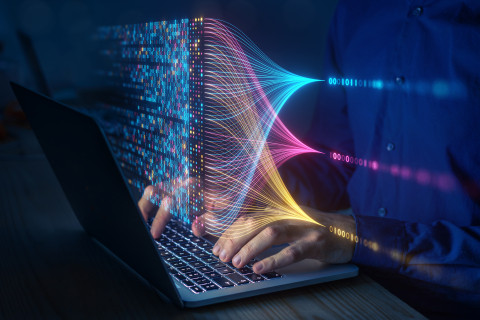
BIFSG for Race Imputation in Colorado: Does it Pass the Test?
Understand the new Colorado regulation as Radost Wenman assesses how BI(F)SG can be used.
Read Now
5 minutes
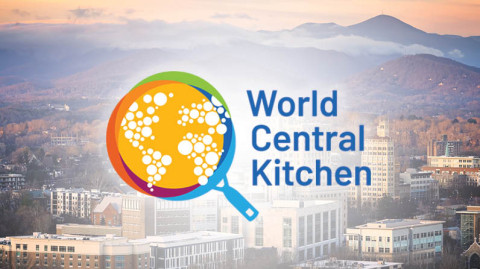
World Central Kitchen: Fighting Hunger in Post-Helene Asheville, One Plate at a Time
Learn more about the World Central Kitchen and their aid in Asheville, North Carolina.
Read Now
3 minutes
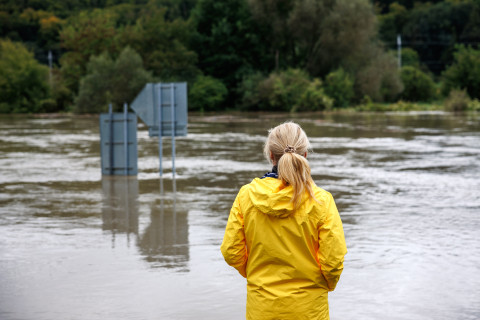
Black Swans and Actuaries
Read more about the major events that impact the industry.
Read Now
5 minutes
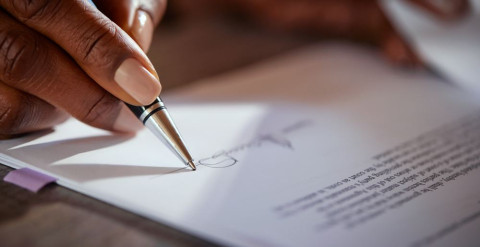
Highlights from the American Academy of Actuaries 2024 Seminar on Effective P/C Loss Reserve Opinions
Read Nicole McArdle's recap of the seminar.
Read Now
3 minutes
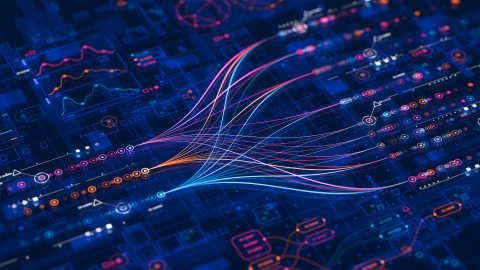
Breaking Down the Concept and Algebra Behind SHAP
Learn more about the tool shedding light on the inner workings of complex algorithmic systems.
Read Now
5 minutes
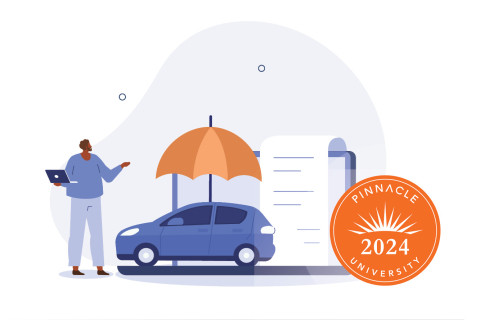
Auto Physical Damage: Pricing, Reserving and Current Trends
A Pinnacle University team looks at the state of the auto physical damage insurance market.
Read Now
6 minutes